by Preventx
Background
One in five free postal test kits ordered through the internet are not returned for laboratory testing after 30 days. This increases with users ordering blood tests or placing an order for the first time. Knowing which users are not likely to return an ordered test kit on time allows Public Health services to take pre-emptive steps to increase returns using a targeted approach. The aim of this study was to develop a predictive model that estimates user return probability using online activity data and machine learning.
Method
Using anonymised triage data from 301,791 users ordering an STI test kit from UK online STI testing service between March 1, 2022, and August 30, 2022, we trained and tuned an ensemble model based on four machine learning algorithms. We assessed accuracy after 25-fold repeated cross-validation and hold-out validation testing. Our final model was further tested for accuracy on user subsets, and on data gathered from a different time period for stability.
Results
Significant variables used in building our final model were derived from users’ online request activity and their previous return history. Interestingly, other strong predictors of returns included age at request, ethnicity and the level of IMD deprivation which was integral for classifying users with no order history. Our final model performed well with an area under the curve (AUC) of 93.5% with an accuracy of 81.8% (kappa = 0.64) on hold-out data. The accuracy of the model reduced slightly to 81.6% (kappa = 0.63) and more significantly to 71.3% (kappa = 0.43) when tested on users ordering blood tests and first-time users respectively. There were no significant changes in performance when tested on data sampled from a different period.
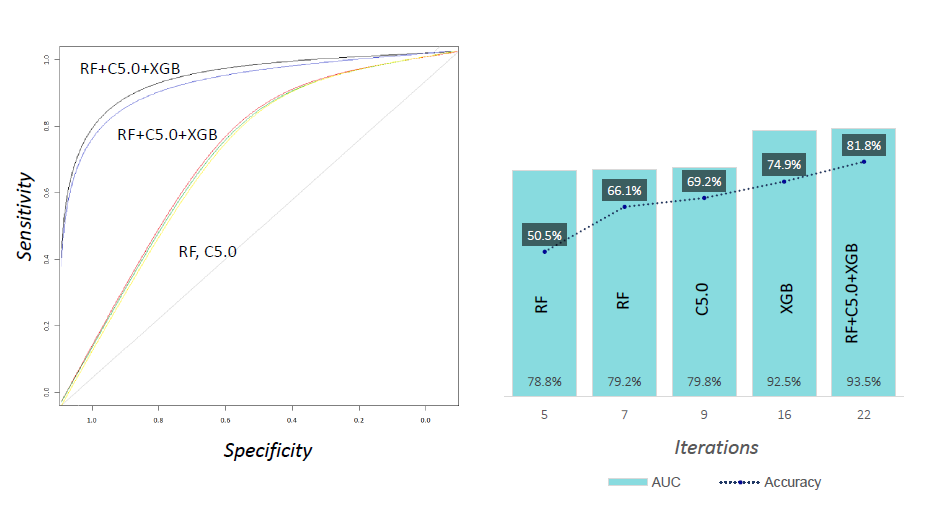
Conclusion
Our model can accurately identify users who won’t return a test kit. Its use of user demographic as well as engagement data increases its robustness for classifying different categories of users. In addition to highlighting how data obtained from users’ request processes can be used to improve outcomes, our study provides information on characteristics and behaviours of users that are predictive of test kit returns.
About the author:
Claire is the Senior Digital Marketing Executive at Preventx.